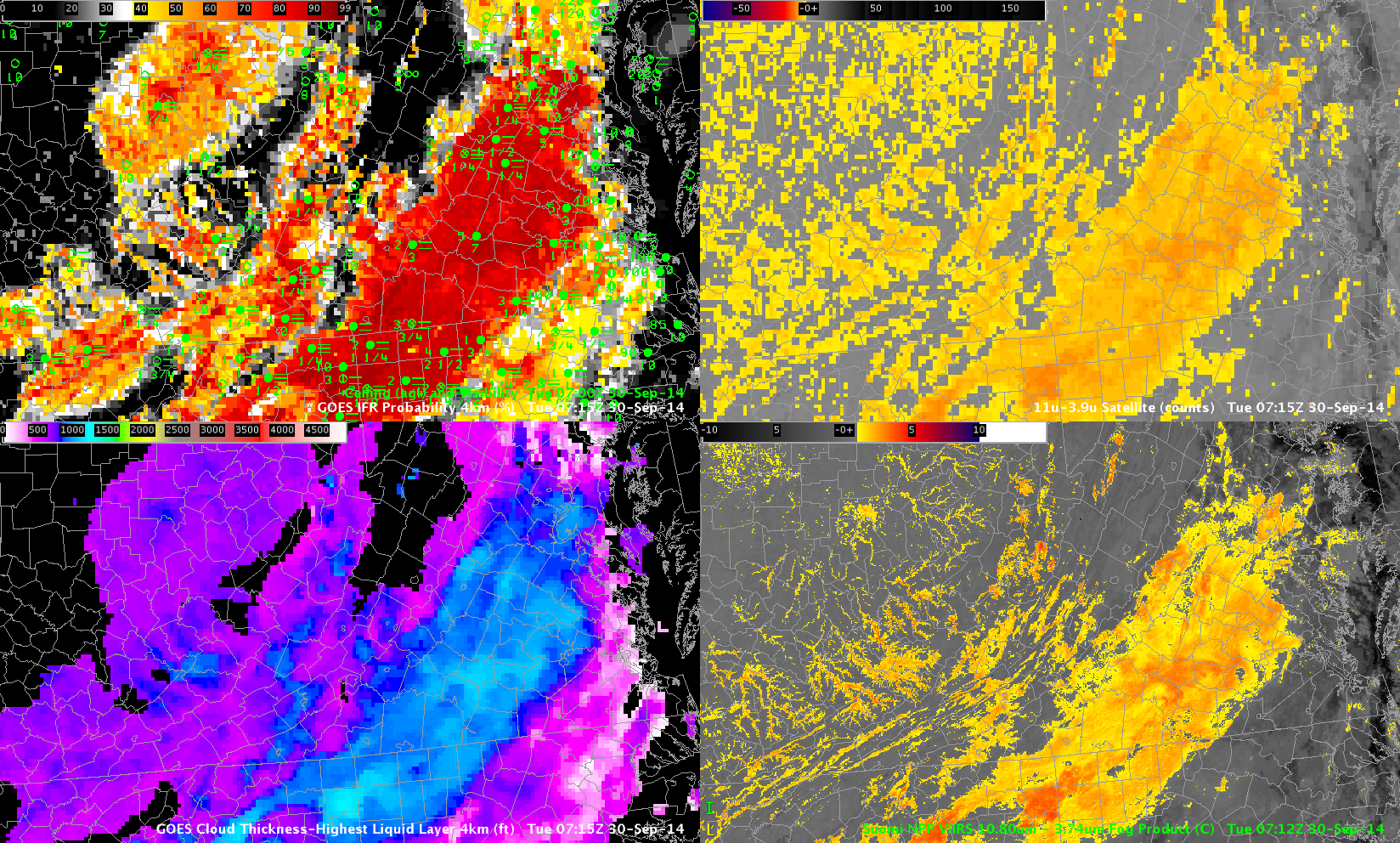
GOES-R IFR Probabilities (Upper Left), GOES-R Cloud Thickness (Lower Left), Brightness Temperature Differences from GOES-13 (Top Right, 10.8 µm – 3.9 µm) and from Suomi-NPP (Bottom Right, 11.35 µm -3.74 µm , all at 0715 UTC 30 September (Click to enlarge)
Radiation fog developed over the Piedmont of Virgina on the morning of 30 September in a region with little pressure gradient. The image above, from 0715 UTC, shows IFR Probability to be very high (from 85-95%) over the Virginia Piedmont. Numerous stations in the region were reporting IFR visibilities and ceilings. Brightness Temperature Difference products are also highlighting the region (Note the advantage in the Suomi NPP Brightness Temperature Difference field that ably captures fine detail related to Ridge/Valley topography over the Appalachians); the advantage of IFR Probability fields is that it includes surface information so that someone using the field can be more certain that the stratus detected by the satellite is in contact with the ground as fog. The animation below shows the evolution of the fields from 0200 through 0700 UTC.
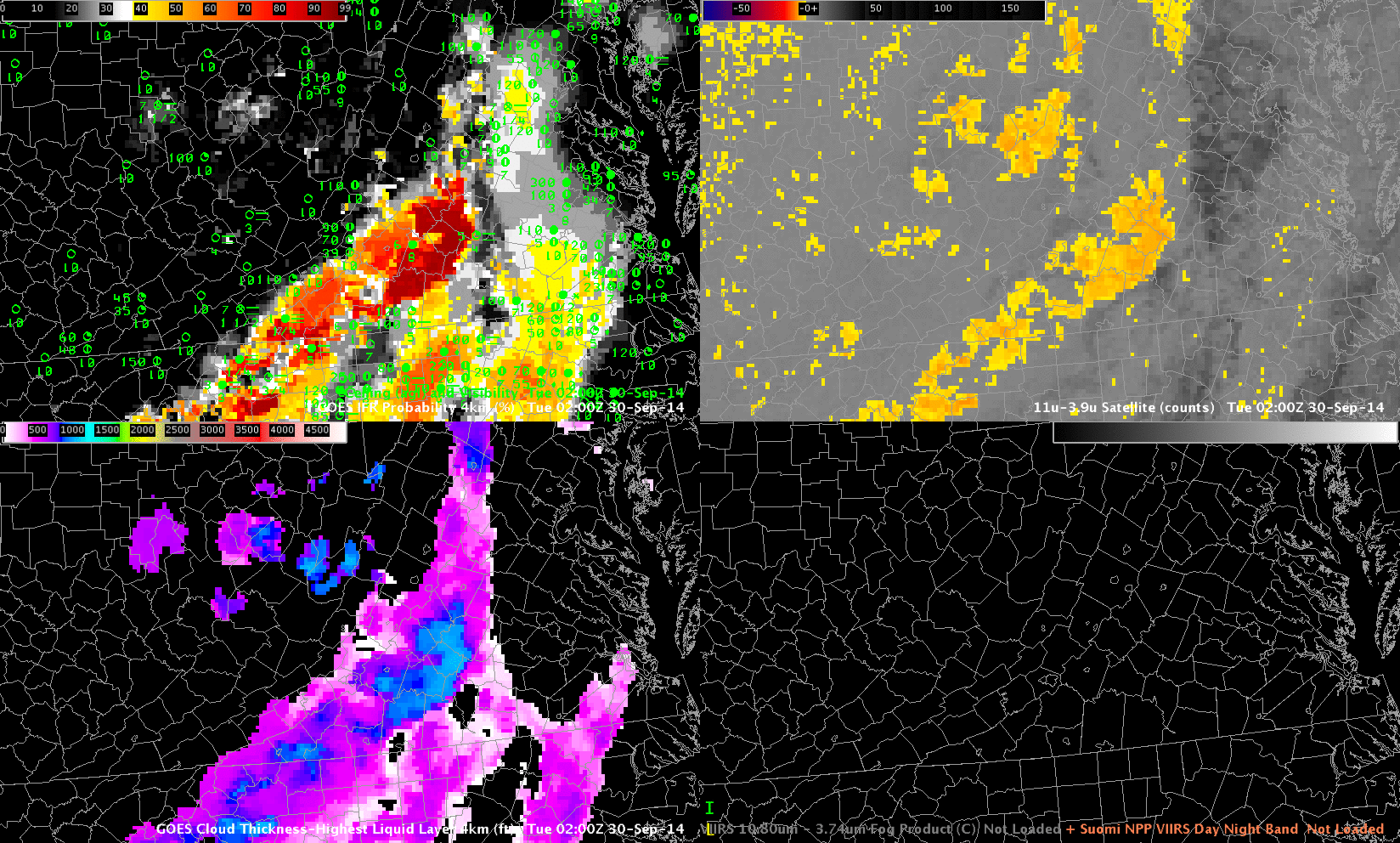
As above, but with Suomi NPP Day/Night band in lower Right. Hourly imagery from 0200 through 0700 UTC on 30 September 2014 (Click to enlarge)
GOES-R Cloud Thickness (the thickness of the highest water-based cloud) can be used to estimate when fog/low clouds will burn off. The estimate is most accurate for strict radiation fog. GOES-R Cloud Thickness is only computed for water-based clouds during non-twilight times (in other words, it is not computed in the hours surrounding twilight at both sunrise and sunset). The last value of Cloud Thickness before morning twilight (shown below) can be used in concert with this scatterplot to guess when clouds might dissipate. Values over eastern Virginia, near Richmond, exceeding 1100 feet, correspond to a dissipation time 4+ hours after 1100 UTC, or at 1500 UTC. In this case, that value was an underestimate.