MODIS data, although infrequent, can give a high-resolution estimate of whether of not fog/low clouds are forming in a region. This is particularly useful for cases with highly variable terrain (such as deep river valleys). In the image above, IFR Probabilities are very low over both the Central Valley of California and over the Salinas Valley closer to the coast. Fog/low Stratus are unlikely to be occurring.
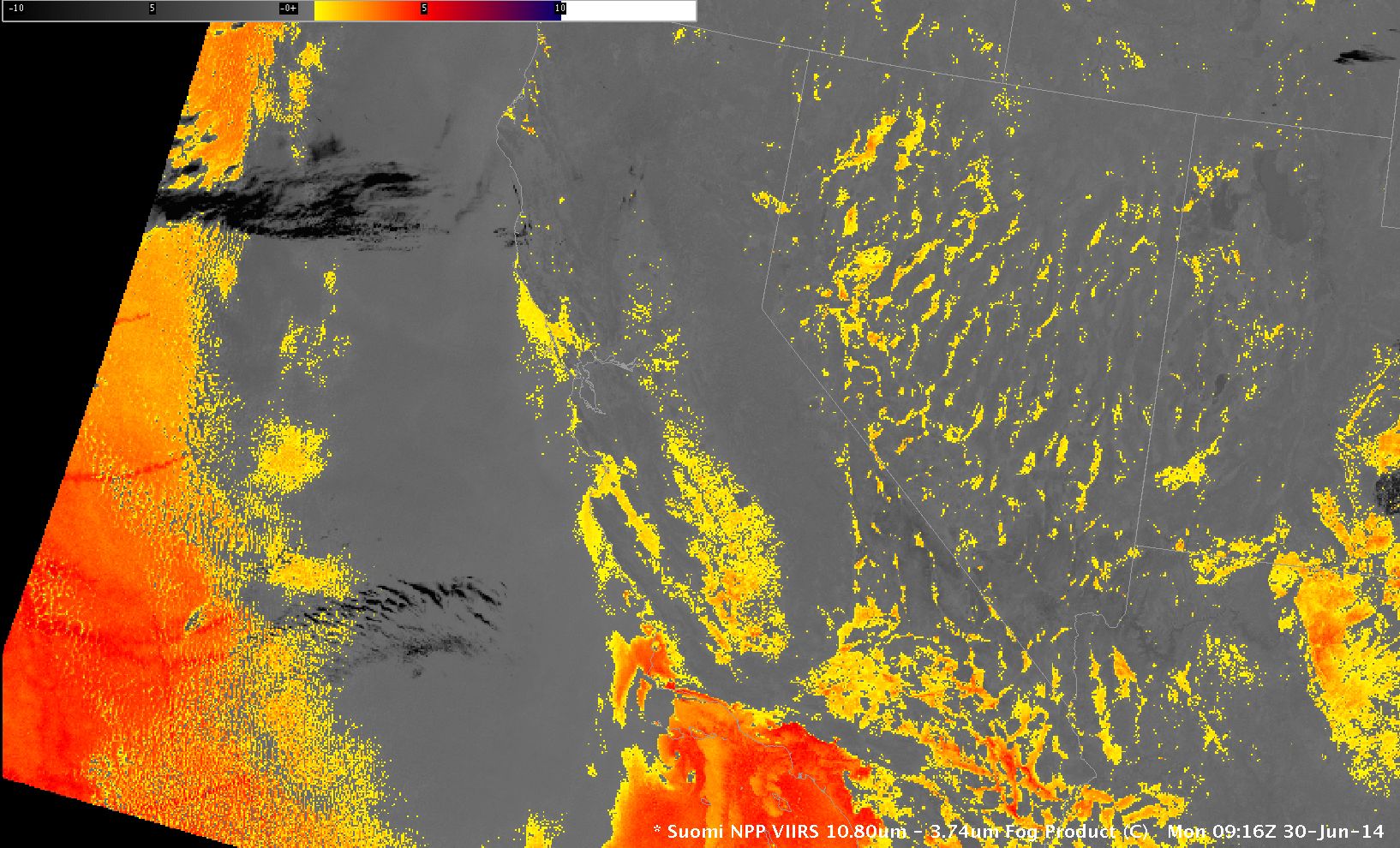
Suomi/NPP Brightness Temperature Difference Fields (11.35µm – 3.74µm) at 0916 and 1057 UTC on 30 June 2014 (Click to enlarge)
The orbital geometry of Suomi/NPP on June 30th was such that it provided two close-up views of these two valleys around/after the time of the MODIS pass shown above. The animation above toggles between those two times. The brightness temperature difference field shows a general increase in return signal strength. But the MODIS IFR Probability field, top, and GOES-West IFR Probability fields (not shown) support the view that any clouds present are not having an impact on ceilings and visibility. A toggle that includes ceilings and visibilities is here.
Note that returns that suggest low clouds over Nevada are more likely due to emissivity differences in different soils, an effect that is more obvious in very dry conditions.
The Suomi/NPP Day/Night band can also provide imagery to help identify the tops of clouds. However, Day/Night Band imagery uses reflected moonlight. There was a new moon on June 27th, and that almost-new moon had set by the time of the images below. Thus, only Earthglow is illuminating any clouds that are present, and that feeble light is mostly overwhelmed by emitted city lights. It is therefore very difficult to identify any changes in cloudcover from the Day/Night band on this date.